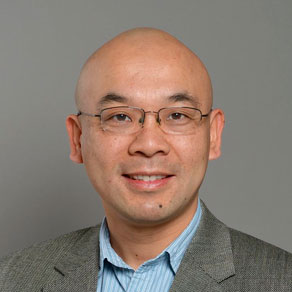
Jianlin Cheng
[introductory/intermediate] Deep Learning for Bioinformatics
Summary
Deep learning has been applied to bioinformatics for more than one decade and is revolutionizing how large amounts of heterogenous biological data are analyzed and how scientific problems are solved. In this course, I will introduce key deep learning methods widely used in bioinformatics and computational biology. Particularly, I will use protein structure prediction as an example to show how deep learning was introduced to the field, how it evolved over years to overcome major scientific challenges, and how it eventually solved one of the top scientific problems through an end-to-end, physics-inspired deep learning architecture – AlphaFold. In addition to protein structure prediction, I will describe how deep learning has been successfully applied to several other key bioinformatics problems such as protein-drug interaction prediction, 3D genome and gene regulation. After completing this course, students will not only understand various deep learning technologies that have been successfully developed and applied in bioinformatics, but also grasp the cutting-edge new deep learning advances such as graph transformer that will revolutionize both bioinformatics and other scientific fields in years down the road.
Syllabus
- Introduction to the Theory of Deep Learning
- Deep Learning for Protein Structure Prediction
- Deep Learning for Drug-Protein Interaction, 3D Genome, and Gene Regulation
- Future Trends and Conclusion
References
None.
Pre-requisites
Basic exposure to statistics, calculus, and/or linear algebra.
Short bio
Dr. Jianlin Cheng is the Thompson Distinguished Professor in the Department of Electrical Engineering and Computer Science at the University of Missouri, Columbia, USA. He earned his PhD in computer science from the University of California, Irvine in 2006. His research is focused on machine learning and bioinformatics. Dr. Cheng has authored or co-authored 171 journal articles (https://scholar.google.com/citations?user=t9MY6lwAAAAJ&hl=en&oi=ao), which have been cited >15,500 times, and he has an h-index of 56. His protein structure prediction method – MULTICOM – was consistently ranked among the top methods in the last seven rounds of Critical Assessments of Protein Structure Prediction (CASP8-14) from 2008 to 2020. His research has been supported by the National Institutes of Health (NIH), National Science Foundation (NSF) and Department of Energy (DoE), USA. Dr. Cheng received an NSF CAREER award in 2012, was recognized as one of top 100 global AI leaders in drug discovery and healthcare by Deep Knowledge Analytics in 2019, and was elected to the American Institute for Medical and Biological Engineering (AIMBE) College of Fellows in 2022. Dr. Cheng currently serves as an associate editor of IEEE/ACM Transactions on Computational Biology and Bioinformatics (TCBB).