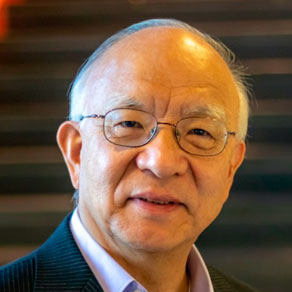
Jiawei Han
[advanced] Text Mining and Deep Learning: Exploring the Power of Pretrained Language Models
Summary
Text embedding and pretrained language models have strongly impacted recent studies in natural language processing and text mining. We overview the major methods on text embedding, deep learning, and pretrained language models related to text mining and present some recent progress on how to apply the concepts and tools of text embedding and pretrained language models in various text mining tasks, including information extraction, taxonomy construction, topic discovery, text classification, and taxonomy-guided text analysis. We show that text embedding and pretrained language models will play a key role at transforming massive text data into structured knowledge.
Syllabus
- An Introduction to Text Embedding and Pre-Trained Language Models
- Weakly Supervised Text Embedding and Embedding-Driven Topic Discovery
- Taxonomy Construction and Enrichment with Pre-Trained Language Models
- Information Extraction Enhanced by Pre-Trained Language Models
- Weakly-Supervised and Taxonomy-Guided Text Classification
- Advanced Text Mining Empowered by Pre-Trained Embeddings
- Summary and Future Directions
References
Blei, D. M., Ng, A. Y., & Jordan, M. I. (2003). Latent dirichlet allocation. Journal of Machine Learning Research.
Clark, K., Luong, M. T., Le, Q. V., & Manning, C. D. (2020). Electra: Pre-training text encoders as discriminators rather than generators. ICLR.
Devlin, J., Chang, M., Lee, K., & Toutanova, K. (2019). BERT: Pre-training of Deep Bidirectional Transformers for Language Understanding. NAACL-HLT.
Xiaotao Gu , Zihan Wang , Zhenyu Bi , Yu Meng, Liyuan Liu, Jiawei Han, Jingbo Shang. “UCPhrase: Unsupervised Context-aware Quality Phrase Tagging.” (KDD’21).
Jingbo Shang, Jialu Liu, Meng Jiang, Xiang Ren, Clare R Voss, and Jiawei Han. 2018. Automated phrase mining from massive text corpora. IEEE Transactions on Knowledge and Data Engineering (2018).
Matthew Honnibal, Ines Montani, Sofie Van Landeghem, and Adriane Boyd. 2020. spaCy: Industrial-strength Natural Language Processing in Python.
Jiaxin Huang, Yiqing Xie, Yu Meng, Yunyi Zhang and Jiawei Han, “CoRel: Seed-Guided Topical Taxonomy Construction by Concept Learning and Relation Transferring”, KDD (2020).
Lan, Z., Chen, M., Goodman, S., Gimpel, K., Sharma, P., & Soricut, R. (2020). Albert: A lite bert for self-supervised learning of language representations. ICLR.
Li, X. L., & Liang, P. (2021). Prefix-tuning: Optimizing continuous prompts for generation. ACL.
Liu, Y., Ott, M., Goyal, N., Du, J., Joshi, M., Chen, D., … & Stoyanov, V. (2019). Roberta: A robustly optimized bert pretraining approach. arXiv preprint arXiv:1907.11692.
Meng, Y., Huang, J., Wang, G., Zhang, C., Zhuang, H., Kaplan, L.M., & Han, J. (2019). Spherical Text Embedding. NeurIPS.
Meng, Y., Huang, J., Wang, G., Wang, Z., Zhang, C., Zhang, Y., & Han, J. (2020). Discriminative topic mining via category-name guided text embedding. WWW.
Meng, Y., Zhang, Y., Huang, J., Zhang, Y., Zhang, C., & Han, J. (2020). Hierarchical topic mining via joint spherical tree and text embedding. KDD.
Meng, Y., Zhang, Y., Huang, J., Zhang, Y., & Han, J. (2022). Topic Discovery via Latent Space Clustering of Pretrained Language Model Representations. WWW.
Meng, Y., Shen, J., Zhang, C., & Han, J. “Weakly-supervised neural text classification”, CIKM’18.
Meng, Y., Zhang, Y., Huang, J., Xiong, C., Ji, H., Zhang, C., & Han, J. “Text Classification Using Label Names Only: A Language Model Self-Training Approach”, EMNLP’20.
Mikolov, T., Sutskever, I., Chen, K., Corrado, G.S., & Dean, J. (2013). Distributed Representations of Words and Phrases and their Compositionality. NIPS.
Schick, T., & Schütze, H. (2021). Exploiting cloze questions for few shot text classification and natural language inference. EACL.
Shen, J., Qiu, W., Meng, Y., Shang, J., Ren, X., & Han, J., “TaxoClass: Hierarchical Multi-Label Text Classification Using Only Class Names”, NAACL’21.
Wang, Z., Mekala, D., & Shang, J. “X-Class: Text Classification with Extremely Weak Supervision”, NAACL’21.
Yang, Z., Dai, Z., Yang, Y., Carbonell, J., Salakhutdinov, R., & Le, Q. V. (2019). XLNet: Generalized Autoregressive Pretraining for Language Understanding. NeurIPS.
Pre-requisites
Basic knowledge on data mining and machine learning; basic knowledge on natural language processing and deep learning.
Short bio
Jiawei Han is Michael Aiken Chair Professor in the Department of Computer Science, University of Illinois at Urbana-Champaign. He received ACM SIGKDD Innovation Award (2004), IEEE Computer Society Technical Achievement Award (2005), IEEE Computer Society W. Wallace McDowell Award (2009), and Japan’s Funai Achievement Award (2018). He is Fellow of ACM and Fellow of IEEE and served as the Director of Information Network Academic Research Center (INARC) (2009-2016) supported by the Network Science-Collaborative Technology Alliance (NS-CTA) program of U.S. Army Research Lab and co-Director of KnowEnG, a Center of Excellence in Big Data Computing (2014-2019), funded by NIH Big Data to Knowledge (BD2K) Initiative. Currently, he is serving on the executive committees of two NSF funded research centers: MMLI (Molecular Make Research Institute)—one of NSF funded national AI centers since 2020— and I-Guide—The National Science Foundation (NSF) Institute for Geospatial Understanding through an Integrative Discovery Environment since 2021.