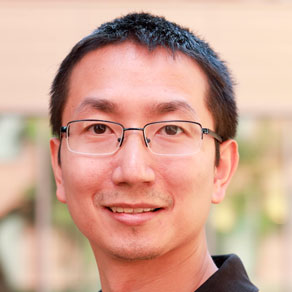
Quanquan Gu
[intermediate/advanced] Benign Overfitting in Machine Learning: From Linear Models to Neural Networks
Summary
In modern machine learning, complex models such as deep neural networks have received increasing popularity. These complicated models are known to be able to fit noisy training data sets, while at the same time achieving small test errors. This benign overfitting phenomenon is not a unique feature of deep learning. Even for linear models and kernel methods, recent work has demonstrated that interpolators on the noisy training data can still perform near optimally on the test data. In this short course, I will talk about a series of recent results on benign overfitting, ranging from minimum-norm interpolator, constant step-size stochastic gradient descent (SGD) to two-layer convolutional neural networks. I will also briefly discuss benign overfitting in adversarial training.
Syllabus
- Benign overfitting in linear regression/ridge regression
- Benign overfitting in stochastic gradient descent
- Benign overfitting in two-layer convolutional neural networks
- Benign overfitting in adversarial training
References
Belkin, M., Ma, S., & Mandal, S. (2018, July). To understand deep learning we need to understand kernel learning. In International Conference on Machine Learning (pp. 541-549).
Hastie, T., Montanari, A., Rosset, S., & Tibshirani, R. J. (2019). Surprises in high-dimensional ridgeless least squares interpolation. arXiv preprint arXiv:1903.08560.
Belkin, M., Hsu, D., & Xu, J. (2020). Two models of double descent for weak features. SIAM Journal on Mathematics of Data Science, 2(4), 1167-1180.
Muthukumar, V., Vodrahalli, K., Subramanian, V., & Sahai, A. (2020). Harmless interpolation of noisy data in regression. IEEE Journal on Selected Areas in Information Theory, 1(1), 67-83.
Bartlett, P. L., Long, P. M., Lugosi, G., & Tsigler, A. (2020). Benign overfitting in linear regression. Proceedings of the National Academy of Sciences, 117(48), 30063-30070.
Tsigler, A. & Bartlett, (2020). Benign overfitting in ridge regression. arXiv preprint arXiv:2009.14286.
Chatterji, N. S., & Long, P. M. (2021). Finite-sample analysis of interpolating linear classifiers in the overparameterized regime. Journal of Machine Learning Research, 22(129), 1-30.
Cao, Y., Gu, Q., & Belkin, M. (2021). Risk bounds for over-parameterized maximum margin classification on sub-gaussian mixtures. Advances in Neural Information Processing Systems, 34.
Zou, D., Wu, J., Braverman, V., Gu, Q., & Kakade, S. M. (2021). Benign Overfitting of Constant-Stepsize SGD for Linear Regression. In COLT.
Wu, J., Zou, D., Braverman, V., Gu, Q., & Kakade, S. M. (2021). Last Iterate Risk Bounds of SGD with Decaying Stepsize for Overparameterized Linear Regression. arXiv preprint arXiv:2110.06198.
Zou, D., Wu, J., Braverman, V., Gu, Q., & Kakade, S. M. (2021). The Benefits of Implicit Regularization from SGD in Least Squares Problems. In NeurIPS.
Zou, D., Wu, J., Braverman, V., Gu, Q., & Kakade, S. M. (2022). Risk Bounds of Multi-Pass SGD for Least Squares in the Interpolation Regime. arXiv preprint arXiv:2203.03159.
Zou, D., Cao, Y., Li, Y., & Gu, Q. (2021). Understanding the Generalization of Adam in Learning Neural Networks with Proper Regularization. arXiv preprint arXiv:2108.11371.
Cao, Y., Chen, Z., Belkin, M., & Gu, Q. (2022). Benign Overfitting in Two-layer Convolutional Neural Networks. arXiv preprint arXiv:2202.06526.
Chen, J., Cao, Y., & Gu, Q. (2021). Benign Overfitting in Adversarially Robust Linear Classification. arXiv preprint arXiv:2112.15250.
Pre-requisites
Linear algebra, calculus, probability theory and statistics, machine learning, optimization.
Short bio
Quanquan Gu is an Assistant Professor of Computer Science at UCLA. His research is in the area of artificial intelligence and machine learning, with a focus on developing and analyzing nonconvex optimization algorithms for machine learning to understand large-scale, dynamic, complex, and heterogeneous data and building the theoretical foundations of deep learning and reinforcement learning. He received his Ph.D. degree in Computer Science from the University of Illinois at Urbana-Champaign in 2014. He received Alfred P. Sloan Research Fellowship and NSF CAREER Award, among many other research awards from industrial companies. He has published 100+ peer-reviewed papers on top machine learning venues such as JMLR, MLJ, COLT, ICML, NeurIPS, AISTATS, UAI. He also serves as an associate/section editor for Journal of Artificial Intelligence and PLOS One, area chair/senior program committee member for ICML, NeurIPS, ICLR, AISTATS, AAAI, UAI and IJCAI.