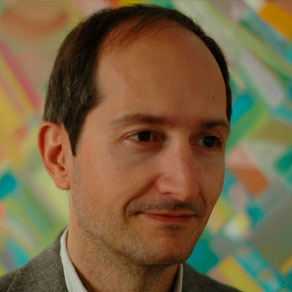
Tommaso Dorigo
Deep-Learning-Optimized Design of Experiments: Challenges and Opportunities
Summary
Take the chain rule of differential calculus, model your system with continuous functions, add overparametrization and an effective way to navigate stochastically through the parameter space in search of an extremum of an utility function, and you have all it takes to find an optimal solution to even the hardest optimization problem. Deep learning, nowadays “differentiable programming”, is boosting our reach to previously intractable problems.
In fundamental physics research, there is an additional complexity – the data-generating processes are stochastic and the models are implicit. This makes them a special and interesting laboratory where to study end-to-end optimization. In this lecture I will look into the challenges of the application of differentiable programming to research in particle physics and related areas, the present status, and I will make a few observations of the possible future developments and opportunities in this area of research.
Short bio
Tommaso Dorigo is an experimental particle physicist who works as a first researcher for the Italian National Institute of Nuclear Physics (INFN). He belongs to the CMS experiment at the CERN Large Hadron Collider and directs the MODE collaboration, which studies differentiable programming applications to fundamental research. He is an expert of statistical data analysis and machine learning techniques. Dorigo is also active in science outreach with a 17-year-old blog, and is an editor of the journals “Reviews in Physics” and “Physics Open”.