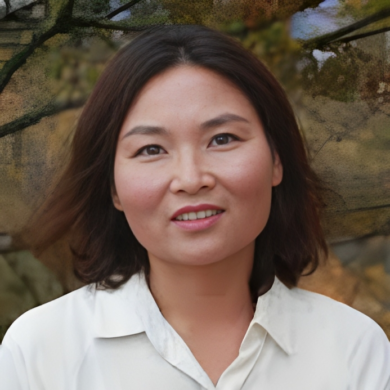
Xinghua Mindy Shi
[introductory/intermediate] Trustworthy Machine Learning for Human Health and Medicine
Summary
Recent biomedical data deluge has fundamentally transformed biomedical research into a data science frontier. The unprecedented accumulation of biomedical data presents a unique yet challenging opportunity to develop novel methods leveraging artificial intelligence and machine learning to further our understanding of biology and advance medicine. In this talk, I will first introduce the cutting-edge research in characterizing human genetic variation and their associations with human health and disease. I will then present recent developments in trustworthy machine learning including our work on trustworthy machine learning covering topics in robustness, interpretability, fairness, security, and privacy of models as well as data.
Syllabus
A brief introduction to human genetics and genomic medicine, introduction to machine learning in biomedicine, as well as trustworthy machine learning covering topics on robustness, interpretability, fairness, security, and privacy.
References
1. Ebert P et al. (2021), “Haplotype-resolved Diverse Human Genomes and Integrated Analysis of Structural Variation”, Science. 0036-8075 (2021). (PMID: 33632895 – PMCID: PMC8026704).
2. Shi X and Wu X (2017), “An Overview of Human Genetic Privacy”, Annals of NY Academy of Sciences, Jan. 1387(1):61-72. (PMID: 27626905 – PMCID: PMC5697154).
3. Varshney KR (2022), “Trustworthy Machine Learning”.
Pre-requisites
Basic knowledge or familiarity with machine learning.
Short bio
Xinghua Mindy Shi is an associate professor at the Department of Computer and Information Sciences and a core faculty member at the Institute for Genomics and Evolutionary Medicine at Temple University. Before that she was an assistant professor at the Department of Bioinformatics and Genomics in the University of North Carolina at Charlotte. She received her Ph.D. and M.S. degrees in Computer Science from the University of Chicago, and postdoctoral training at Brigham and Women’s Hospital, Harvard Medical School, and Broad Institute. With research interests across the intersection of computer science and biomedical data science, her group is focused on developing statistical and machine learning tools to solve large-scale computational problems in biology and medicine. Her general research areas include bioinformatics, data science, and trustworthy artificial intelligence. Dr. Shi is a recipient of the NSF CAREER award and a distinguished member of the Association for Computing Machinery (ACM).