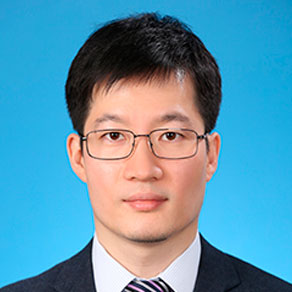
Peng Cui
[intermediate/advanced] Stable Learning for Out-of-Distribution Generalization: Invariance, Causality and Heterogeneity
Summary
The traditional framework of machine learning (ML) operates under the assumption that training and testing datasets are independent and identically distributed (i.i.d.). This assumption, however, often proves inadequate in real-world scenarios where distributional shifts between training and test data can significantly impair model performance post-deployment. Such phenomena underscore the critical importance of addressing the Out-of-Distribution (OOD) generalization problem, an emerging topic of ML research that focuses on scenarios wherein the test distributions differ from the training ones.
This course aims to provide a comprehensive view on the stable learning framework, which aims to enhance the model’s OOD generalization ability from three perspectives, including invariance, causality, and heterogeneity. Invariance is at the core of stable learning, which seeks invariant prediction mechanisms that hold across different domains / distributions. This course will share the recent progress as well as the drawbacks of invariant learning. Then, we will move on to the causality, serving as a foundation of invariance from causal inference. The course will introduce essential concepts, methodologies, and the latest advancements of causality, and demonstrate how feature decorrelation can lead to the generalization out of distribution.
Beyond model-centric strategies, this course will delve into heterogeneity-aware ML, as another way to pursue invariance by leveraging the “variance” within data. This data-centric approach aims to enhance generalization under distributional shifts by modeling and leveraging data heterogeneity throughout the whole ML pipeline. Attendees will learn about the types of data heterogeneity, alongside quantitative metrics and algorithms that consider heterogeneous data. Real-world applications, including healthcare, autonomous control systems (like self-driving cars), and finance, will serve as practical examples throughout the course.
Finally, we will discuss the potential directions in this field, covering understanding real-world distribution shifts, scaling methods to large language models (LLMs) / foundation models, and benchmarking OOD generalization capabilities.
At the end of the course, attendees will gain a comprehensive understanding of OOD generalization’s foundational principles, including key methodologies, recent developments, limitations, and exciting prospects for future research in the field.
Syllabus
- Background: performance degradation of ML models in real-world applications, the causes of poor OOD generalization performances.
- OOD generalization problem: the problem setting of OOD generalization, differences with related fields, typical methodologies.
- Stable learning: framework, core ideas.
- Invariance: concepts, recent progress, and drawbacks in practice.
- Causality: essential concepts, methodologies, and the latest advancements.
- Heterogeneity: quantitative metrics, typical algorithms built on heterogeneous data, benchmarks.
- Future directions: the pattern of distribution shifts, OOD generalization benchmarks.
References
Cui, P., & Athey, S. (2022). Stable learning establishes some common ground between causal inference and machine learning. Nature Machine Intelligence, 4(2), 110-115.
Shen, Z., Cui, P., Zhang, T., & Kuang, K. (2020, April). Stable learning via sample reweighting. In Proceedings of the AAAI Conference on Artificial Intelligence (Vol. 34, No. 04, pp. 5692-5699).
Zhang, X., Cui, P., Xu, R., Zhou, L., He, Y., & Shen, Z. (2021). Deep stable learning for out-of-distribution generalization. In Proceedings of the IEEE/CVF Conference on Computer Vision and Pattern Recognition (pp. 5372-5382).
Liu, J., Hu, Z., Cui, P., Li, B., & Shen, Z. (2021, July). Heterogeneous risk minimization. In International Conference on Machine Learning (pp. 6804-6814). PMLR.
Liu, J., Wu, J., Pi, R., Xu, R., Zhang, X., Li, B., & Cui, P. (2022, September). Measure the Predictive Heterogeneity. In The Eleventh International Conference on Learning Representations.
Liu, J., Wang, T., Cui, P., & Namkoong, H. (2024). On the need for a language describing distribution shifts: Illustrations on tabular datasets. Advances in Neural Information Processing Systems, 36.
Pre-requisites
General machine learning knowledge.
Short bio
Peng Cui is an Associate Professor with tenure in Tsinghua University. He is interested in research on stable prediction, decision-making based on causal principles, and network representation learning at a large scale. Since 2016, he has been exploring how to combine causal statistics with machine learning methods, and developed a theoretical framework for stable learning inspired by causality. His research results have been widely used in industrial domains such as intelligent health care and the Internet economy. He has published more than 100 papers in top artificial intelligence conferences and received 7 awards for his papers from international conferences or journals. He is an associate editor of international journals such as IEEE TKDE, ACM TOMM, ACM TIST, IEEE TBD, KAIS, etc., and has been area chair or senior PC member of top conferences like NeurIPS, ICML, UAI, etc. He has won the second prize of the National Natural Science Award in China, the first prize of the Natural Science Award of the Ministry of Education in China, the CCF-IEEE CS Young Scientist Award, and he is a distinguished member of the ACM.