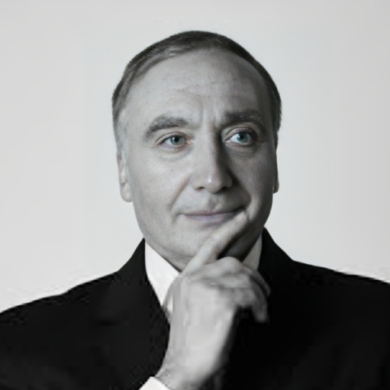
Pierre Baldi
[intermediate/advanced] From Deep Learning and Transformers to AI Risks and Safety
Summary
First these lectures will provide an introduction to the history and theory behind AI and deep learning. Then the focus will shift to the many applications of deep learning to the natural sciences, by demonstrating how deep learning methods can be used to tackle beautiful and fundamental problems in physics, chemistry, and biomedicine. Examples of problems that will be considered include the search for exotic particles and dark matter, the prediction of molecular properties and chemical reactions, and the classification and understanding of images and videos in biomedical imaging. We will then study transformers, attention mechanisms, large language models, and their applications. And finally we will examine some of the likely future trends and related ethical and societal issues and discuss possible solutions. Throughout the lectures particular emphasis will be given to first-principles thinking.
Syllabus
1. Historical Introduction. Theory of Deep Learning. The Quest for How the Brain Learns.
2. Applications of Deep Learning in Physics and Chemistry.
3. Applications of Deep Learning in BioMedicine: the AI-driven Hospital of the Future.
4. Transformers, Large Language Models, Attention Mechanisms and their Applications.
5. Future Trends and Challenges: Technical, Ethical, Economic, and Societal Frontiers.
References
P. Baldi. Deep Learning in Science. Cambridge University Press, 2021.
Pre-requisites
Basic calculus, algebra, and probability theory. Some familiarity with machine learning and neural networks is preferable.
Short bio
Pierre Baldi earned MS degrees in Mathematics and Psychology from the University of Paris, and a PhD in Mathematics from the California Institute of Technology. He is currently Distinguished Professor in the Department of Computer Science, Founding Director of the AI in Science Institute, and Associate Director of the Center for Machine Learning and Intelligent Systems at the University of California Irvine. The long term focus of his research is on understanding intelligence in brains and machines. He has made several contributions to the theory of AI and deep learning, and developed and applied AI and deep learning methods for the natural sciences, to address problems in physics, chemistry, and bio-medicine. He recently published his fifth book: Deep Learning in Science, Cambridge University Press (2021). His honors include the 1993 Lew Allen Award at JPL, the 2010 E. R. Caianiello Prize for research in machine learning, the 2023 Dennis Gabor Award of the International Neural Network Society, and election to Fellow of the AAAS, AAAI, IEEE, ACM, and ISCB. He serves as Associated Editor for Artificial Intelligence, Neural Networks, and the IEEE/ACM Transactions in Computational Biology and Bioinformatics. He has mentored ~100 graduate students and postdoctoral fellows and co-founded several startup companies. At UCI, he has introduced several new courses, including the course AI Frontiers: Technical, Ethical, and Societal.