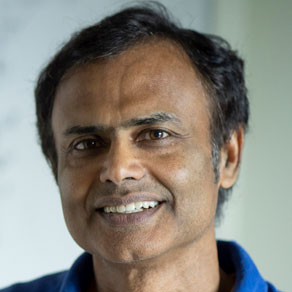
Kaushik Roy
[intermediate] Re-engineering Computing with Neuro-inspired Learning: Algorithms, Architecture, and Devices
Summary
Advances in machine learning, notably deep learning, have led computers to match or surpass human performance in several cognitive tasks including vision, speech and natural language processing. However, implementation of neural algorithms in conventional “von-Neumann” architectures are several orders of magnitude more area and power expensive than the biological brain. Hence, we need fundamentally new approaches to sustain the exponential growth in performance at high energy-efficiency. Exploring the new paradigm of computing necessitates a multi-disciplinary approach: exploration of new learning algorithms inspired from neuroscientific principles, developing network architectures best suited for such algorithms, new hardware techniques to achieve orders of improvement in energy consumption, and nanoscale devices that can closely mimic the neuronal and synaptic operations. In this talk, I will present our recent work on spike-based learning to achieve high energy efficiency with accuracy comparable to that of standard analog deep-learning techniques. Input coding from DVS cameras has been used to develop energy efficient hybrid SNN/ANN networks for optical flows, gesture recognition, and language translation. Additionally, we propose probabilistic neural and synaptic computing platforms that can leverage the underlying stochastic device physics of spin-devices. System-level simulations indicate ~100x improvement in energy consumption for such spintronic implementations over a corresponding CMOS implementation across different computing workloads. Complementary to the above device efforts, we have explored different local/global learning algorithms including stochastic learning with one-bit synapses that greatly reduces the storage/bandwidth requirement while maintaining competitive accuracy, and adaptive online learning that efficiently utilizes the limited memory and resource constraints to learn new information without catastrophically forgetting already learnt data.
Syllabus
- Basics of neuromorphic computing; input representations
- Training large scale spiking and non-spiking networks – local and global learning
- Spiking networks for solving beyond perception centric tasks
- Hardware implications and design of neuromorphic in-memory computing hardware for energy efficiency
References
Selected references – will add more later
- K. Roy, P. Panda, A. Jaiswal, “Towards spike-based machine intelligence with neuromorphic computing,” Nature 575 (7784) 607-617.
- A. Sengupta et. al. ,” Going deeper in spiking neural networks: Vgg and residual architectures,” Frontiers in neuroscience 13.
- E.O. Neftci, H. Mostafa, F. Zenke, “Surrogate gradient learning in spiking neural networks: Bringing the power of gradient-based optimization to spiking neural networks,” IEEE Signal Processing Magazine 36 (6), 51-63.
- N. Rathi and K. Roy, “DIET-SNN: A Low-Latency Spiking Neural Network With Direct Input Encoding and Leakage and Threshold Optimization”, IEEE Transactions on Neural Networks and Learning Systems.
Pre-requisites
Basics of regression and neural networks, basic computer architecture and logic design.
Short bio
Kaushik Roy is the Edward G. Tiedemann, Jr., Distinguished Professor of Electrical and Computer Engineering at Purdue University. He received his BTech from Indian Institute of Technology, Kharagpur, PhD from University of Illinois at Urbana-Champaign in 1990 and joined the Semiconductor Process and Design Center of Texas Instruments, Dallas, where he worked for three years on FPGA architecture development and low-power circuit design. His current research focuses on cognitive algorithms, circuits and architecture for energy-efficient neuromorphic computing/ machine learning, and neuro-mimetic devices. Kaushik has supervised 91 PhD dissertations and his students are well placed in universities and industry. He is the co-author of two books on Low Power CMOS VLSI Design (John Wiley & McGraw Hill).
Dr. Roy received the National Science Foundation Career Development Award in 1995, IBM faculty partnership award, ATT/Lucent Foundation award, 2005 SRC Technical Excellence Award, SRC Inventors Award, Purdue College of Engineering Research Excellence Award, Outstanding Mentor Award in 2021, Humboldt Research Award in 2010, 2010 IEEE Circuits and Systems Society Technical Achievement Award (Charles Desoer Award), IEEE TCVLSI Distinguished Research Award in 2021, Distinguished Alumnus Award from Indian Institute of Technology (IIT), Kharagpur, Fulbright-Nehru Distinguished Chair, DoD Vannevar Bush Faculty Fellow (2014-2019), Semiconductor Research Corporation Aristotle award in 2015, and best paper awards at 1997 International Test Conference, IEEE 2000 International Symposium on Quality of IC Design, 2003 IEEE Latin American Test Workshop, 2003 IEEE Nano, 2004 IEEE International Conference on Computer Design, 2006 IEEE/ACM International Symposium on Low Power Electronics & Design, 2005 and 2019 IEEE Circuits and system society Outstanding Young Author Award (Chris Kim, Abhronil Sengupta), 2006 IEEE Transactions on VLSI Systems best paper award, 2012 ACM/IEEE International Symposium on Low Power Electronics and Design best paper award, 2013 IEEE Transactions on VLSI Best paper award. Dr. Roy was a Purdue University Faculty Scholar (1998-2003). He was a Research Visionary Board Member of Motorola Labs (2002) and held the M. Gandhi Distinguished Visiting faculty at Indian Institute of Technology (Bombay) and Global Foundries visiting Chair at National University of Singapore. He has been in the editorial board of IEEE Design and Test, IEEE Transactions on Circuits and Systems, IEEE Transactions on VLSI Systems, and IEEE Transactions on Electron Devices. He was Guest Editor for Special Issue on Low-Power VLSI in the IEEE Design and Test (1994) and IEEE Transactions on VLSI Systems (June 2000), IEE Proceedings — Computers and Digital Techniques (July 2002), and IEEE Journal on Emerging and Selected Topics in Circuits and Systems (2011). Dr. Roy is a fellow of IEEE.