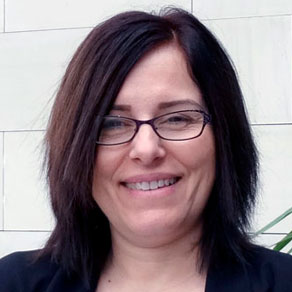
Lina J. Karam
[introductory/intermediate] Deep Learning for Quality Robust Visual Recognition
Summary
Computer vision systems are increasingly relying on machine learning with deep neural networks (DNNs) for various applications ranging from entertainment, manufacturing, and security to healthcare, mobility, and retail. While deep neural networks (DNNs)perform on par with – or better than – humans on pristine high-resolution images, DNN performance is significantly worse than human performance on images with quality degradations, which are frequently encountered in real-world applications. DNNs were also shown to be very sensitive to small adversarial perturbation even when the perceived visual quality of the images is not affected. This tutorial first presents fundamental concepts in machine learning and deep learning with focus on computer vision applications. It then presents a selective review of visual quality factors and adversarial perturbations that were found to significantly affect the DNN recognition performance along with strategies for increasing the resilience of DNNs in real-world environments.
Syllabus
The first part of the tutorial will cover introductory fundamental concepts in machine learning and deep learning with a focus on computer vision and deep neural networks (DNNs). The second part will focus on factors that affect the DNN recognition performance in computer vision applications including image quality factors and adversarial attacks. The third part will cover select strategies for increasing the robustness of DNNs in real-world environment.
References
[1] I. Goodfellow and Y. Bengio and A. Courville, Deep Learning, MIT Press, 2016. FREE Access at http://www.deeplearningbook.org/
[2] S. Dodge and L. Karam, Introduction to Machine Learning and Deep Learning: A Hands-On Starter’s Guide. FREE Access at http://www.deeplearningtextbook.org/
[3] S. F. Dodge and L. J. Karam, “Understanding How Image Quality Affects Deep Neural Networks,” International Conference on the Quality of Multimedia Experience (QoMEX), 6 pages, June 2016. doi: 10.1109/QoMEX.2016.7498955
[4] S. Dodge and L. Karam, “A Study and Comparison of Human and Deep Learning Recognition Performance Under Visual Distortions,” 7 pages, International Conference on Computer Communications and Networks (ICCCN), July-Aug. 2017.
[5] S. Dodge and L. Karam, “Can the Early Human Visual System Compete with Deep Neural Networks?,” 7 pages, International Conference on Computer Vision (ICCV), Workshop on Mutual Benefits of Cognitive and Computer Vision (MBCC), Oct. 2017. Oral Presentation.
[6] L. J. Karam, T. Borkar, J. Chae, Y. Cao, “Generative Sensing: Transforming Unreliable Data for Reliable Recognition,” IEEE Multimedia Information Processing and Retrieval (IEEE MIPR), Apr. 2018.
[7] S. F. Dodge and L. J. Karam, “Quality Robust Mixtures of Deep Neural Networks,” IEEE Transactions on Image Processing, vol. 27, no. 11, pp. 5553-5562, Nov. 2018.
[8] S.F. Dodge and L.J. Karam, “Human and DNN Classification Performance on Images With Quality Distortions: A Comparative Study,” ACM Transactions on Applied Perception, vol. 16, issue 2, 18 pages, March 2019; doi 10.1145/3306241.
[9] T.S. Borkar and L.J. Karam, “DeepCorrect: Correcting DNN Models against Image Distortions,” IEEE Transactions on Image Processing, vol. 28, issue 12, pp. 6022-6034, Dec. 2019.
[10] T. Borkar, F. Heide, and L.J. Karam, “Defending Against Universal Attacks through Selective Feature Regeneration,” IEEE Conference on Computer Vision and Patten Recognition (CVPR), 11 pages, June 2020.
[11] L.J. Karam and T. Borkar, “Systems and Methods for Feature Corrections and Regeneration for Robust Sensing, Computer Vision, and Classification,” United States Patent US11,0304,85. Issued June 2021.
[12] Y. Deng and L.J. Karam, “Universal adversarial attack via enhanced projected gradient descent,” IEEE International Conference on Image Processsing, pages 1241-1245, October 2020.
[13] Y. Deng and L.J. Karam, “Frequency-Tuned Universal Adversarial Perturbations,” European Conference on Computer Vision Workshops, pages 494-510, August 2020.
[14] Y. Deng and L.J. Karam, “Towards Imperceptible Universal Attacks on Texture Recognition,” arXiv:2011.11957, Nov. 2020. Available Online at: https://arxiv.org/abs/2011.11957
[15] Y. Deng and Li.J. Karam, “A Study for Universal Adversarial Attacks on Texture Recognition,” arXiv:2010.01506, Oct. 2020. Available Online at: https://arxiv.org/abs/2010.01506
[16] S.-M. Moosavi-Dezfooli et al. “Universal adversarial perturbations.” CVPR, 2017.
[17] E. Rodner et al. “Fine-grained recognition in the noisy wild: sensitivity analysis of convolutional neural networks approaches.” arXiv, 2016.
[18] I. Vasiljevic et al. “Examining th.e impact of blur on recognition by convolutional networks,” arxiv, 2016.
[19] Y. Zhou et al. “On classification of distorted images with deep convolutional neural network.” arXiv, 2017.
[20] S. Zheng et al. “Improving the robustness of deep neural networks via stability training.” arXiv, 2016.
[21] Z. Sun et al. “Feature quantization for defending against distortion of images.” CVPR, 2018.
[22] Z. Diamond et al. “Dirty pixels: Optimizing image classification architectures for raw sensor data.” arXiv, 2017.
[23] S.T. Chen et al. “ShapeShifter: Robust Physical Adversarial Attack on Faster R-CNN Object Detector.” arXiv, 2019.
[24] I. Goodfellow et al. “Explaining and harnessing adversarial examples.” arxiv, 2014; ICLR, 2015.
[25] A. Kurakin et al. “Adversarial machine learning at scale.” ICLR, 2017.
[26] K.R. Mopuri et al. “NAG: Network for adversary generation.” CVPR, 2018.
[27] O. Poursaeed et al. “Generative adversarial perturbations.” CVPR, 2018.
[28] A. Athalye. Et al. “Synthesizing robust adversarial examples.” ICML, 2018.
[29] T.B. Brown et al. “Adversarial patch.” arXiv, 2018.
[30] K. Eykholt et al. “Robust Physical-World Attacks on Deep Learning Visual Classification,” CVPR, 2018.
[31] L. Karam and T. Borkar, “Systems and methods for feature transformation, correction and regeneration for robust sensing, transmission, computer vision, recognition, and classification,” US Patent 11,030,485. Issued June 2021.
Pre-requisites
Mathematics at the level of an undergraduate degree in engineering or computer science (calculus, probability theory, and linear algebra).
Short bio
Prof. Lina J. Karam is the Dean of the School of Engineering and Professor of Electrical and Computer Engineering at the Lebanese American University. She is an IEEE Fellow and Editor-In-Chief of the IEEE Journal on Selected Topics in Signal Processing (IEEE JSTSP). Prior to joining LAU, Prof. Karam was a Full Professor in the School of Electrical, Computer & Energy Engineering, Arizona State University, where she also served as Computer Engineering Director for Industry Engagement and where she currently holds a Professor Emerita position. Prof. Karam was awarded a U.S. National Science Foundation CAREER Award, a NASA Technical Innovation Award, IEEE Region 6 Award, IEEE SPS Best Paper Award, Intel Outstanding Researcher Award, and IEEE Phoenix Section Outstanding Faculty Award. Prof. Karam’s industrial experience includes video compression R&D at AT&T Bell Labs, multidimensional data processing and visualization at Schlumberger, and collaborations on computer vision, machine learning, image/video processing, compression, and transmission projects with various industries. She served as General Chair of IEEE ICIP 2016 and as General Co-Chair of IEEE ICME 2019. She helped initiate the World’s First Visual Innovation Award that was presented for the first time at IEEE ICIP 2016 and more recently the World’s First Multimedia Star Innovator Award that was presented at IEEE ICME 2019. Prof. Karam has over 250 technical publications and she is an inventor on 8 issued US patents. She served on the IEEE Publication Services and Products Board (PSPB) Strategic Planning Committee, IEEE SPS Board of Governors, IEEE CAS Fellow Evaluation Committee, and the IEEE SPS Conference and Award Boards. In addition to serving as EiC of IEEE JSTSP, Prof. Karam is currently serving on the IEEE TechRxiv Advisory Board, IEEE Access Journal Editorial Board, IEEE SPS Awards, Conference and Publications Boards. She is also an expert delegate of the ISO/IEC JTC1/SC29 Committee (Coding of audio, picture, multimedia and hypermedia information) and participating in JPEG/MPEG standardization activities.