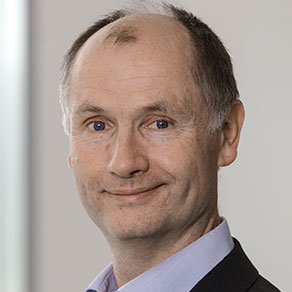
Martin Schultz
[intermediate] Deep Learning for Air Quality, Weather and Climate
Summary
The environment we live in is a complex system with manifold feedbacks across a wide range of spatial and temporal scales. Environmental sensors generate massive amounts of data every day, and numerical models are employed around the world to predict air quality, weather, and climate. It seems clear that deep learning, which has been so successful in areas such as image or speech recognition or robotics, will have a huge potential to improve our current predictive capabilities, or at the least to achieve similar results at much cheaper computational cost. Indeed, several groups are now actively researching the use of deep learning for improved data handling (e.g. in data assimilation), as a surrogate for empirical model parameterisations, or for the detection of patterns in model output. However, the properties of environmental data and the chaotic behaviour of the climate system impose large challenges on deep learning approaches and render many highly successful deep learning methods unsuitable for application in this area. The course will begin with an introduction to weather and climate from a data science perspective. We will briefly summarize the history of machine learning in this area. In the second half, we will investigate the relations between environmental data properties and various deep learning approaches and discuss some of the more sophisticated deep learning studies for air quality, weather and climate.
Syllabus
Part 1: Introduction to weather and climate from a data science perspective
Part 2: Statistical and technical properties of environmental (i.e. atmospheric) data
Part 3: Machine learning versus numerical modelling of air quality, weather and climate
Part 4: Current research into deep learning for air quality, weather and climate
References
- Reichstein M, Camps-Valls G, Stevens B, Jung M, Denzler J, Carvalhais N, et al. 2019. Deep learning and process understanding for data-driven earth system science. Nature 566(7743), 195–204. (doi:10.1038/s41586-019-0912-1)
- Schultz M. G., Betancourt C., Gong B., Kleinert F., Langguth M., Leufen L. H., Mozaffari A. and Stadtler S. (2021): Can deep learning beat numerical weather prediction? Phil. Trans. R. Soc. A.3792020009720200097. http://doi.org/10.1098/rsta.2020.0097
Pre-requisites
Good knowledge of basic machine learning, some knowledge of deep learning methods (CNN, Auto-encoder, GAN, LSTM). Basic knowledge of statistics.
Short bio
Martin Schultz is a senior researcher at the Jülich Supercomputing Centre. After a successful career as environmental scientist developing air pollution and chemistry climate models, he started a new line of research to investigate how deep learning can be used to improve the analysis and predictions of air quality, weather and climate. Through the ERC Advanced Grant IntelliAQ which was awarded to him in 2018, and through several other research projects Schultz built a 20-member team of atmospheric scientists, computer scientists and mathematicians, who adopt state-of-the-art deep learning models for atmospheric applications, develop new approaches, and analyse the fundamental properties of the data and the models in order to understand why certain methods work better than others and where the theoretical limitations of deep learning may be. His research group also develops scalable, performant machine learning workflows and software packages and is involved in the creation of benchmark datasets to help boost deep learning developments in environmental research. Schultz has been listed twice as highly cited researcher and has given several courses on atmospheric science and, more recently, machine learning.