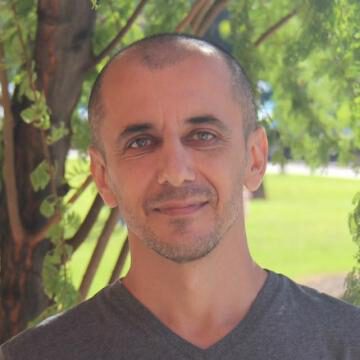
Mihai Surdeanu
Explainable Deep Learning for Natural Language Processing
Summary
While deep learning approaches to natural language processing (NLP) have had many successes, they can be difficult to understand, augment, or maintain as needs shift. In this talk I will discuss two recent efforts that aim to bring explainability back into deep learning methods for NLP.
In the first part of the talk, I will introduce an explainable approach for information extraction (IE), an important NLP task, which mitigates the tension between generalization and explainability by jointly training for the two goals. Our approach uses a multi-task learning architecture, which jointly trains a classifier for information extraction, and a sequence model that labels words in the context of the relation that explain the decisions of the relation classifier. This sequence model is trained using a hybrid strategy: supervised, when supervision from pre-existing patterns is available, and semi-supervised otherwise. In the latter situation, we treat the sequence model’s labels as latent variables, and learn the best assignment that maximizes the performance of the extractor. We show that, even with minimal guidance for what makes a good explanation, i.e., 5 rules per relation type to be extracted, the sequence model provides labels that serve as accurate explanations. Further, we show that the joint training generally improves the performance of the IE classifier.
In the second part of the talk, we adapt recent advances from the adjacent field of program synthesis to information extraction, synthesizing extraction rules directly from a few provided examples. We use a transformer-based architecture to guide an enumerative search, and show that this reduces the number of steps that need to be explored before a rule is found. Further, we show that without training the synthesis algorithm on the specific domain, our synthesized rules achieve state-of-the-art performance in a 1-shot IE task, i.e., when only 1 example is provided for each class to be learned.
Short bio
Dr. Surdeanu works on NLP systems that process and extract meaning from natural language texts such as question answering (answering natural language questions), information extraction (converting free text into structured relations and events), and textual entailment. He focuses mostly on interpretable models, i.e., approaches where the computer can explain in human understandable terms why it made a decision, and machine reasoning, i.e., methods that approximate the human capacity to understand bigger things from knowing smaller facts. He published more than 100 peer-reviewed articles, including four articles that were among the top three most cited articles at their respective venues that year. His work has been cited more than 15 thousand times, and has a current h-index of 41. Dr. Surdeanu’s work was funded by several United States government organizations (DARPA, NIH, NSF), as well as private foundations (the Allen Institute for Artificial Intelligence, the Bill Melinda Gates Foundation).