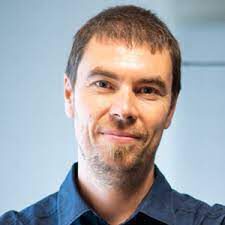
Yvan Saeys
[introductory/intermediate] Interpreting Machine Learning Models
Summary
In this series of lectures we will cover a wide range of topics related to the interpretation of machine learning models. While some machine learning models can be easily interpreted, other models are more black boxes, and in general there is a trade-off between the predictive performance and the ease of interpretation of machine learning models. To cope with this, many techniques have been introduced to shed light on the inner workings of machine learning models and how to extract insights from them.
We will start by a general introduction on machine learning interpretability, relating it also to trustworthiness of machine learning models. We will discuss the different types of interpretability methods, including model-based and post-hoc interpretability methods as well as model distillation techniques.
Next we will cover several interpretability methods in detail, starting with model-based techniques, followed by post-hoc interpretability methods (including LIME and SHAP) and instance based explanations (including counterfactuals). A large part of the lectures will also be devoted to feature attribution techniques to interpret deep learning models (including class activation maps, DeepLift, and several gradient based methods). In between the theory underlying the methods, examples on real world data sets will be used to illustrate the different interpretability methods.
Syllabus
- Introduction, general context, and overview of the field
- Model based interpretability methods
- Post-hoc interpretability methods
- Interpreting deep learning models
- Model distillation
- Evaluating interpretability methods
- Case studies on real world data sets
References
- Christoph Molnar. Interpretable Machine Learning. A Guide for Making Black Box Models Explainable.
- F. Doshi-Velez and B. Kim, “Towards a rigorous science of interpretable machine learning,” 2017, arXiv:1702.08608.
- G. Hinton, O. Vinyals, and J. Dean (2015). Distilling the Knowledge in a Neural Network. NIPS Deep Learning and Representation Learning Workshop. https://arxiv.org/abs/1503.02531
- Ribeiro, M., Singh, S. & Guestrin, C., 2016. “Why Should I Trust You?”: Explaining the Predictions of Any Classifier. In Proceedings of the 2016 Conference of the North American Chapter of the Association for Computational Linguistics: Demonstrations. Available at: http://dx.doi.org/10.18653/v1/n16-3020
- Murdoch WJ, Singh C, Kumbier K, Abbasi-Asl R, Yu B. Definitions, methods, and applications in interpretable machine learning. Proceedings of the National Academy of Sciences of the United States of America. 2019 Oct;116(44):22071-22080. DOI: 10.1073/pnas.1900654116. PMID: 31619572; PMCID: PMC6825274.
- R. R. Selvaraju, M. Cogswell, A. Das, R. Vedantam, D. Parikh and D. Batra, “Grad-CAM: Visual Explanations from Deep Networks via Gradient-Based Localization,” 2017 IEEE International Conference on Computer Vision (ICCV), 2017, pp. 618-626, doi: 10.1109/ICCV.2017.74.
Pre-requisites
Familiarity with basic machine learning problems (classification, regression, clustering, dimensionality reduction) and approaches (linear models, decision trees, Random Forests, neural networks).
Short bio
Yvan Saeys received a PhD in computer science from Ghent University. After spending time abroad at the University of the Basque Country and the University Claude Bernard Lyon, he returned to Belgium, where he is currently a professor in computer science at the Department of Applied Mathematics, Computer Science and Statistics of Ghent University, and a principal investigator at the Flemish Biotechnology Institute (VIB) where he is heading the “Data Mining and Modeling for Biomedicine” group. His research interests include robustness and interpretability of machine learning methods, and their application to computational biology and medicine.
At Ghent University he teaches several machine learning related courses, including Artificial Intelligence, Machine Learning, Big Data Science and Data Mining. He has published more than 180 papers in computer science and computational biology journals, is serving on the editorial board of the journal “Information Fusion”, and has been reviewing for many computer science and biotechnology journals.
The methods he developed obtained the best results in several machine learning competitions, including winner of the DREAM5 Network Inference challenge, the most important benchmark for assessing machine learning techniques for systems biology, and the best algorithm in the FlowCAP IV challenge on predicting HIV to AIDS progression. He was recently nominated as “Highly cited researcher” in the Cross-Field category (Multiple highly-cited papers that rank in the top 1% by citations, as analyzed by Clarivate Analytics) and received the FWO/AstraZeneca Foundation Award “Patient care in the AI era”.