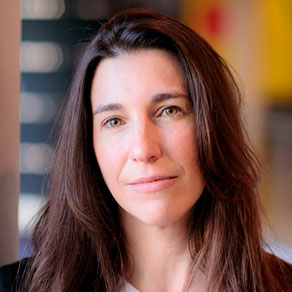
Clarisa Sánchez
[introductory/intermediate] Mechanisms for Trustworthy AI in Medical Image Analysis and Healthcare
Summary
Imaging is a cornerstone of many medical specialties, such as Radiology, Pathology and Ophthalmology. Humans assessing the images are becoming the bottleneck, as they have to read an ever-growing number of more extensive imaging studies. Computers can potentially extract more information from images, more reliably, and more accurately. AI, and particularly deep learning, has so far brought substantial improvements to the analysis of medical images, reaching and even surpassing human performance in many cases. However, is this technology ready to be trusted with our health decisions? In this course we will explore how AI is transforming healthcare, from detection and diagnosis to treatment monitoring, but also the challenges and state-of-the-art mechanisms to accomplish trustworthy AI solutions that can be adopted in clinical practice.
Syllabus
- Deep learning and medical image analysis: General overview
- Trustworthy AI: concepts and guidelines
- State-of-the-art mechanisms for bias identification, domain adaptation and explainability in medical imaging
- State-of-the-art mechanisms for robustness to malicious attacks in medical imaging
- Benchmarking and validation of AI systems for medical image analysis
- Regulatory and privacy considerations of medical AI
References
Litjens, G., Kooi, T., Bejnordi, B.E., Setio, A.A.A., Ciompi, F., Ghafoorian, M., Van Der Laak, J.A., Van Ginneken, B. and Sánchez, C.I., 2017. A survey on deep learning in medical image analysis. Medical Image Analysis, 42, pp.60-88.
Zhou, S. K., Greenspan, H., Davatzikos, C., Duncan, J. S., Van Ginneken, B., Madabhushi, A., Prince, J. L., Rueckert, D., & Summers, R. M. (2021). A Review of Deep Learning in Medical Imaging: Imaging Traits, Technology Trends, Case Studies with Progress Highlights, and Future Promises. Proceedings of the IEEE, 109(5), 820-838. [9363915].
World Health Organization, 2021. Ethics and governance of artificial intelligence for health: WHO guidance. Accessed: 2021, Dec 12. https://www.who.int/publications/i/item/9789240029200.
European Commission, 2019. Ethics guidelines for trustworthy AI. Accessed: 2021, Dec 22. https://digital-strategy.ec.europa.eu/en/library/ethics-guidelines-trustworthy-ai.
González-Gonzalo, C., Thee, E.F., Klaver, C.C., Lee, A.Y., Schlingemann, R.O., Tufail, A., Verbraak, F. and Sánchez, C.I., 2021. Trustworthy AI: Closing the gap between development and integration of AI systems in ophthalmic practice. Progress in Retinal and Eye Research.
Bortsova, G., González-Gonzalo, C., Wetstein, S.C., Dubost, F., Katramados, I., Hogeweg, L., Liefers, B., van Ginneken, B., Pluim, J.P., Veta, M. and Sánchez, C.I., 2021. Adversarial attack vulnerability of medical image analysis systems: Unexplored factors. Medical Image Analysis, 73, p.102141.
Muehlematter, U.J., Daniore, P. and Vokinger, K.N., 2021. Approval of artificial intelligence and machine learning-based medical devices in the USA and Europe (2015–20): a comparative analysis. The Lancet Digital Health, 3(3), pp.e195-e203.
Pre-requisites
Basic knowledge of machine learning and deep learning for computer vision.
Short bio
Clarisa Sánchez Gutiérrez (PhD Telecommunication Engineering, 2008) is a Professor of AI and Health at the University of Amsterdam. Double appointment at the Faculty of Science (Informatics Institute) and at Amsterdam University Medical Centers. Her research aims at enhancing patient care by designing and enabling responsible AI technologies in healthcare. Research focus on developing, validating, and clinically integrating AI solutions to solve medical image analysis challenges encountered in different steps of the patient pathway: from prevention and triage, through diagnosis and decision making, to care delivery and management. 100+ publications; 11,000+ citations (Google Scholar). Organizer and program chair of the first MIDL conference. Organizing and program committee member of international conferences, workshops and challenges, such as MICCAI, MIDL, ISBI, Med-NeurIPS. Associate Editor of Medical Imaging Analysis. Co-inventor of CAD4TB solution currently used in clinical practice. Scientific director of ICAI labs Thira Lab and ICAI AI for Oncology Lab. President of the Dutch Society of Pattern Recognition and Image Processing.