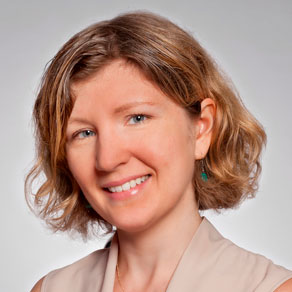
Kate Saenko
Overcoming Dataset Bias in Deep Learning [virtual]
Summary
In machine learning, “dataset bias” happens when the training data is not representative of future test data. Finite datasets cannot include all variations possible in the real world, so every machine learning dataset is biased in some way. Yet, machine learning progress is traditionally measured by testing on in-distribution data. This obscures the real danger that models will fail on new domains. For example, a pedestrian detector trained on pictures of people in the sidewalk could fail on jaywalkers. A medical classifier could fail on data from a new sensor or hospital. The good news is, we can fight dataset bias with techniques from domain adaptation, semi-supervised learning and generative modeling. I will describe the evolution of efforts to improve domain transfer, their successes and failures, and some practical advice.
Short bio
Kate is an Associate Professor of Computer Science at Boston University and a consulting professor for the MIT-IBM Watson AI Lab. She leads the Computer Vision and Learning Group at BU, is the founder and co-director of the Artificial Intelligence Research (AIR) initiative, and member of the Image and Video Computing research group. Kate received a PhD from MIT and did her postdoctoral training at UC Berkeley and Harvard. Her research interests are in the broad area of Artificial Intelligence with a focus on dataset bias, adaptive machine learning, learning for image and language understanding, and deep learning.