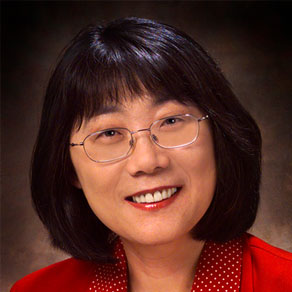
Aidong Zhang
Concept-Based Explainable Deep Learning Models
Summary
The past decade has been a very exciting time for deep learning (DL) research. Significant research effort has focused on improving predictive performance of Deep Neural Networks (DNN) by proposing increasingly complex architectures which have surpassed even human-level performance. Even though these methods demonstrate incredible potential in saving valuable man-hours and minimizing inadvertent human mistakes, their adoption has been met with rightful skepticism and extreme circumspection in critical applications like medical diagnosis, credit risk analysis, etc. The most paramount of these challenges is the lack of rationale behind DNN predictions – making them notoriously black-box in nature. In extreme cases, this can create a lack of alignment between the designer’s intended behavior and the model’s actual performance. Recently, there have been multiple explainable deep learning model architectures proposed. In this talk, I will discuss some of the latest techniques on robustness and interpretability of DL approaches. In particular, I will discuss the concept-based deep learning models and how generalizable concepts can be learned.
Short bio
Dr. Aidong Zhang is a Professor of Computer Science in the School of Engineering and Applied Sciences at University of Virginia (UVA). She also holds joint appointments with the Department of Biomedical Engineering and School of Data Science at University of Virginia. Her research interests include machine learning, data mining, bioinformatics, and health informatics. She has authored over 380 research publications in these areas. Dr. Zhang is a fellow of ACM, AIMBE, and IEEE.