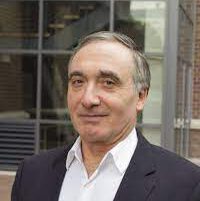
Pierre Baldi
[intermediate/advanced] Deep Learning in Science
Summary
First these lectures will provide an introduction to the history and theory behind deep learning. Then the focus will shift to the many applications of deep learning to the natural sciences, by demonstrating how deep learning methods can be used to tackle beautiful and fundamental problems in physics, chemistry, and biomedicine. Examples of problems that will be considered include the search for exotic particles and dark matter, the prediction of molecular properties and chemical reactions, and the classification and understanding of images and videos in biomedical imaging. Some of the likely future trends and related ethical and societal issues will be discussed at the end.
Syllabus
- Historical Introduction. Theory of Deep Learning. The Quest for How the Brain Learns.
- Applications of Deep Learning in Physics and Chemistry.
- Applications of Deep Learning in BioMedicine: the AI-driven Hospital of the Future.
- Future Trends and Technical, Ethical, Economic, and Societal Frontiers.
References
P. Baldi. Deep Learning in Science. Cambridge University Press, 2021.
Pre-requisites
Basic calculus, algebra, and probability theory. Some familiarity with machine learning and neural networks is preferable.
Short bio
Pierre Baldi earned MS degrees in Mathematics and Psychology from the University of Paris, and a PhD in Mathematics from the California Institute of Technology. He is currently Distinguished Professor in the Department of Computer Science, Director of the Institute for Genomics and Bioinformatics, and Associate Director of the Center for Machine Learning and Intelligent Systems at the University of California Irvine. The long term focus of his research is on understanding intelligence in brains and machines. He has made several contributions to the theory of AI and deep learning, and developed and applied AI and deep learning methods for the natural sciences, to address problems in physics (e.g exotic particle detection), chemistry (e.g. reaction prediction), and bio-medicine (e.g. biomedical imaging analysis). He recently published his fifth book: Deep Learning in Science, Cambridge University Press (2021). His honors include the 1993 Lew Allen Award at JPL, the 2010 E. R. Caianiello Prize for research in machine learning, and the election to Fellow of the AAAS, AAAI, IEEE, ACM, and ISCB.