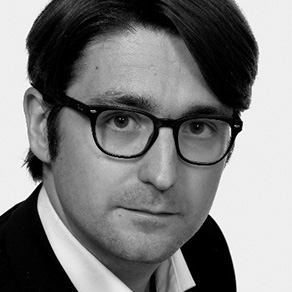
Gregor Kasieczka
[introductory/intermediate] Deep Learning Fundamental Physics: Rare Signals, Unsupervised Anomaly Detection, and Generative Models
Summary
This course will introduce aspects of unsupervised (and weakly supervised) learning methods and demonstrate these concepts using concrete problems from particle physics. After a short introduction to the scientific enviroment, we explore anomaly detection as a potential strategy to search for previously unseen physical processes in a model independent way. We encounter different solutions to this problem, ranging from autoencoders to methods based on density estimation.
The availability of high-quality synthetic data from Monte Carlo (MC) simulation is a key ingredient for the success of particle physics. However, the production and storage of these MC simulations occupies a large fraction of computing resources of big experimental collaborations. We will introduce generative machine learning models such as generative adversarial networks (GANs), variational autoencoders (VAEs), and flow based models which promise a way to greatly speed-up simulation.
Syllabus
- Introduction to the scientific environment in particle physics: Open questions, challenges, and typical data
- Discussion of anomaly detection: Point vs Group Anomalies, Methods for unsupervised anomaly detection
- Overview of generative machine learning models and their application to physics data
References
Deep Learning for Physics Research (https://doi.org/10.1142/12294)
Pre-requisites
Basic knowledge of deep learning and particle physics helpful, but not required.
Short bio
Gregor Kasieczka is an Assistant Professor for machine learning in particle physics at the University of Hamburg. His research includes searching for exotic new theories of physics with the CMS experiment at CERN and developing machine learning algorithms with a focus on particle physics applications. Topics of interest are particle classification, uncertainty estimation and robustness, building generative models for fast simulation, and developing new approaches for the unsupervised detection of anomalies in data.