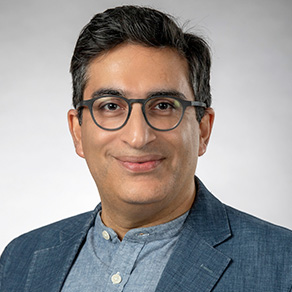
Nitesh Chawla
[introductory/intermediate] Graph Representation Learning
Summary
Complex systems, such as web, information, or knowledge systems, are generally represented as (heterogeneous) networks, which are rich in their representation of the underlying characteristics and phenomena of the complex system. These representations include relationships, attributes, content, and temporal information. As such the networks are inherently heterogeneous (or multi-modal), presenting a significantly large exploratory space for manual feature construction for several downstream modeling tasks. This has led to development and popularization of representation learning algorithms for graphs / networks. In this tutorial, we will answer the following questions: What is representation learning on graphs? Why do we need it? How do we do it? How do we tackle the challenges of multiple data modalities? What are some of the applications?
Syllabus
- Complex Systems as Graphs / Networks
- Overview of Representation Learning
- Learning node-based Embeddings: Homogeneous and Heterogeneous Methods
- Graph Neural Networks
References
1) Cui, P., Wang, X., Pei, J., & Zhu, W. (2018). A survey on network embedding. IEEE Transactions on Knowledge and Data Engineering, 31(5), 833-852.
2) Hamilton, W. L., Ying, R., & Leskovec, J. (2017). Representation learning on graphs: Methods and applications. arXiv preprint arXiv:1709.05584.
3) Liu, X., & Tang, J. (2021). Network representation learning: A macro and micro view. AI Open, 2, 43-64.
4) Dong, Y., Chawla, N. V., & Swami, A. (2017, August). metapath2vec: Scalable representation learning for heterogeneous networks. In Proceedings of the 23rd ACM SIGKDD international conference on knowledge discovery and data mining (pp. 135-144).
5) Zhang, C., Song, D., Huang, C., Swami, A., & Chawla, N. V. (2019, July). Heterogeneous graph neural network. In Proceedings of the 25th ACM SIGKDD International Conference on Knowledge Discovery & Data Mining (pp. 793-803).
6) Saebi, M., Ciampaglia, G. L., Kaplan, L. M., & Chawla, N. V. (2020). HONEM: learning embedding for higher order networks. Big Data, 8(4), 255-269.
Pre-requisites
Introductory machine learning, specifically learning on graphs, and network science.
Short bio
Nitesh Chawla is the Frank M. Freimann Professor of Computer Science and Engineering, and Founding Director of the Lucy Family Institute for Data and Society. His research is focused on machine learning, data science, and network science, and is motivated by the question of how technology can advance the common good through interdisciplinary research. He is the recipient of the IEEE CIS Outstanding Early Career Award; the IBM Watson Faculty Award, the IBM Big Data, and Analytics Faculty Award, National Academy of Engineering New Faculty Fellowship, and 1st Source Bank Technology Commercialization Award. In recognition of the societal and community impact of his research, he received the Rodney F Ganey Award and Michiana 40 under 40 honor. He is founder of Aunalytics, a data science software and cloud computing company.