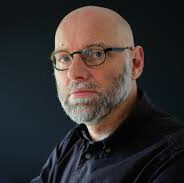
Luc De Raedt
[introductory/intermediate] From Statistical Relational to Neuro-Symbolic Artificial Intelligence
Summary
A central challenge to contemporary AI is to integrate learning and reasoning. The integration of learning and reasoning has been studied for decades already in the fields of statistical relational artificial intelligence and probabilistic programming. StarAI has focussed on unifying logic and probability, the two key frameworks for reasoning, and has extended these probabilistic logics with machine learning principles.
Neuro-Symbolic AI takes a similar perspective but focusses on marrying logical reasoning and neural networks instead.
I will provide an introduction to those two fields and argue that StarAI and Probabilistic Logics form an ideal basis for developing neuro-symbolic artificial intelligence techniques. Thus Neuro-Symbolic AI = StarAI + Neural Networks.
Syllabus
- Introduction to Statistical Relational AI
- Key frameworks in Statistical Relational AI
- Learning and Inference in StarAI
- From StarAI to NeSy — similarities
- Some Neuro Symbolic AI frameworks
References
Luc De Raedt, Kristian Kersting, Sriraam Natarajan, David Poole. Statistical Relational Artificial Intelligence: Logic, Probability, and Computation, Morgan Claypool 2016.
Giuseppe Marra, Sebastijan Dumančić, Robin Manhaeve, Luc De Raedt. From Statistical Relational to Neural Symbolic Artificial Intelligence: A Survey, arXiv preprint arXiv:2108.11451
Luc De Raedt, Angelika Kimmig. Probabilistic (logic) programming concepts, Machine Learning, 2015.
Pre-requisites
Elementary knowledge of logic and graphical models is helpful but not required.
Short bio
Luc De Raedt is full professor at the Department of Computer Science, KU Leuven, and director of Leuven.AI, the newly founded KU Leuven Institute for AI. He is a guestprofessor at Örebro University in the Wallenberg AI, Autonomous Systems and Software Program. He received his PhD in Computer Science from KU Leuven (1991), and was full professor (C4) and Chair of Machine Learning at the Albert-Ludwigs-University Freiburg, Germany (1999-2006). His research interests are in Artificial Intelligence, Machine Learning and Data Mining, as well as their applications. He is well known for his contributions in the areas of learning and reasoning, in particular for his work on probabilistic and inductive programming. He co-chaired important conferences such as ECMLPKDD 2001 and ICML 2005 (the European and International Conferences on Machine Learning), ECAI 2012 and IJCAI-ECAI in 2022 (the European and international AI conferences). He is on the editorial board of Artificial Intelligence, Machine Learning and the Journal of Machine Learning Research. He is an EurAI and AAAI fellow, an IJCAI Trustee and received and ERC Advanced Grant in 2015.