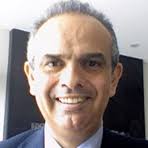
Fabio Roli
[introductory/intermediate] Adversarial Machine Learning
Summary
Today machine-learning algorithms are used for many real-world applications, including image recognition, spam filtering, malware detection, biometric recognition. In these applications, the learning algorithm can have to face intelligent and adaptive attackers who can carefully manipulate data to purposely subvert the learning process. As machine learning algorithms have not been originally designed under such premises, they have been shown to be vulnerable to well-crafted attacks, including test-time evasion and training-time poisoning attacks (also known as adversarial examples). This course aims to introduce the fundamentals of the security of machine learning, the related field of adversarial machine learning, and some techniques to assess the vulnerability of machine-learning algorithms and to protect them from adversarial attacks. We report application examples including object recognition in images, biometric identity recognition, spam and malware detection.
Syllabus
- Introduction to adversarial machine learning: introduction by practical examples from computer vision, biometrics, spam, malware detection.
- Design of learning-based pattern classifiers in adversarial environments. Modelling adversarial tasks. The two-player model (the attacker and the classifier). Levels of reciprocal knowledge of the two players (perfect knowledge, limited knowledge, knowledge by queries and feedback). The concepts of security by design and security by obscurity.
- System design: vulnerability assessment and defense strategies. Attack models against machine learning. Vulnerability assessment by performance evaluation. Taxonomy of possible defense strategies.
- Summary and outlook. Current state of this research field and future perspectives.
References
1. B. Battista, F. Roli. Wild patterns: Ten years after the rise of adversarial machine learning. Pattern Recognition 84 (2018): 317-331.
2. Biggio, B., Corona, I., Maiorca, D., Nelson, B., Srndic, N., Laskov, P., Giacinto, G., Roli, F. Evasion attacks against machine learning at test time. ECML-PKDD, 2013.
3. Biggio, B., Fumera, G., Roli, F. Security evaluation of pattern classifiers under attack. IEEE Trans. Knowl. Data Eng., 26 (4):984-996, 2014.
4. B. Biggio, F.Roli, Wild Patterns, Half-day Tutorial on Adversarial Machine Learning: https://www.pluribus-one.it/research/sec-ml/wild-patterns
Pre-requisites
No knowledge of the course topics is assumed. A basic knowledge of machine learning and statistical pattern classification is requested.
Short bio
Fabio Roli is a Full Professor of Computer Engineering at the University of Genova, Italy, and Founding Director of the Pattern Recognition and Applications laboratory at the University of Cagliari (https://pralab.diee.unica.it/). He is partner of the company Pluribus One that he co-founded (https://www.pluribus-one.it). He has been doing research on the design of pattern recognition and machine learning systems for thirty years. He has provided seminal contributions to the fields of multiple classifier systems and adversarial machine learning, and he has played a leading role in the establishment and advancement of these research themes. He has been appointed Fellow of the IEEE and Fellow of the International Association for Pattern Recognition. He is a recipient of the Pierre Devijver Award for his contributions to statistical pattern recognition.