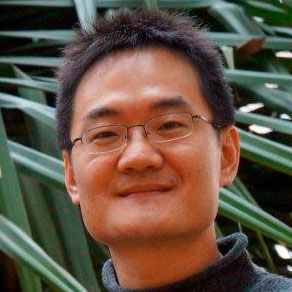
Ming Yuan
[intermediate/advanced] Low Rank Tensor Methods in High Dimensional Data Analysis
Summary
Large amount of multidimensional data in the form of multilinear arrays, or tensors, arise routinely in modern applications from such diverse fields as chemometrics, genomics, physics, psychology, and signal processing among many others. At the moment, our ability to generate and acquire them has far outpaced our ability to effectively extract useful information from them. There is a clear demand to develop novel statistical methods, efficient computational algorithms, and fundamental mathematical theory to analyze and exploit information in these types of data. In these lectures, I will review some of the recent progresses and discuss some of the present challenges.
Syllabus
- Tensor decomposition
- Tensor SVD/PCA
- Tensor completion
- Tensor regression
References
Auddy, A and Yuan, M. (2021), On estimating rank-one spiked tensors in the presence of heavy tailed errors, arXiv preprint arXiv:2107.09660.
Auddy, A and Yuan, M. (2020), Perturbation bounds for orthogonally decomposable tensors and their applications in high dimensional data analysis, arXiv preprint arXiv:2007.09024.
Xia, D. and Yuan, M. (2021), Effective Tensor Sketching via Sparsification, IEEE Transactions on Information Theory, 67(2), 1356-1369.
Luo, Y., Raskutti, G., Yuan, M. and Zhang, A. (2021) A sharp blockwise tensor perturbation bound for orthogonal iteration, Journal of Machine Learning Research, 22(179), 1-48.
Xia, D., Yuan, M. and Zhang, C-H. (2021), Statistically Optimal and Computationally Efficient Low Rank Tensor Completion from Noisy Entries, The Annals of Statistics, 49(1), 76-99.
Zhang, A., Luo, Y., Raskutti, G. and Yuan, M. (2020), ISLET: Fast and Optimal Low-rank Tensor Regression via Importance Sketching, SIAM Journal on Mathematics of Data Science, 2(2), 444-479.
Xia, D. and Yuan, M. (2019), On Polynomial Time Methods for Exact Low Rank Tensor Completion, Foundations of Computational Mathematics, 19(6), 1265-1313.
Raskutti, G., Yuan, M. and Chen, H. (2019), Convex Regularization for High-Dimensional Multi-Response Tensor Regression, The Annals of Statistics, 47(3), 1554-1584.
Chen, H., Raskutti, G. and Yuan, M. (2019), Non-Convex Projected Gradient Descent for Generalized Low-Rank Tensor Regression, Journal of Machine Learning Research, 20(1), 172- 208.
Yuan, M. and Zhang, C-H. (2017), Incoherent Tensor Norms and Their Applications in Higher Order Tensor Completion, IEEE Transactions on Information Theory, 63(10), 6753-6766.
Yuan, M. and Zhang, C.H. (2016), On Tensor Completion via Nuclear Norm Minimization, Foundations of Computational Mathematics, 16(4), 1031-1068.
Pre-requisites
Basic knowledge of machine learning, optimization, computational complexity, multivariate analysis and concentration inequalities.
Short bio
Ming Yuan is Professor of Statistics at Columbia University, and an affiliated investigator at Morgridge Institute for Research. He was previously Senior Investigator in Virology at Morgridge Institute for Research and Professor of Statistics at University of Wisconsin at Madison, and prior to that Coca-Cola Junior Professor of Industrial and Systems Engineering at Georgia Institute of Technology. His research and teaching interests lie broadly in statistics and its interface with other quantitative and computational fields such as optimization, machine learning, computational biology and financial engineering. He has over 100 scientific publications in applied mathematics, computer science, electrical engineering, financial econometrics, medical informatics, optimization, and statistics among others. He served as the program secretary of the Institute for Mathematical Statistics (IMS), and a member of the advisory board for the Quality, Statistics and Reliability (QSR) section of the Institute for Operations Research and the Management Sciences (INFORMS). He was a co-Editor of The Annals of Statistics and has been serving on numerous editorial boards. He was named a Medallion Lecturer of IMS in 2018, and a recipient of the John van Ryzin Award (2004; International Biometrics Society), CAREER Award (2009; US National Science Foundation), the Guy Medal in Bronze (2014; Royal Statistical Society), and the Leo Breiman Junior Researcher Award (2017; the Statistical Learning and Data Mining section of the American Statistical Association).