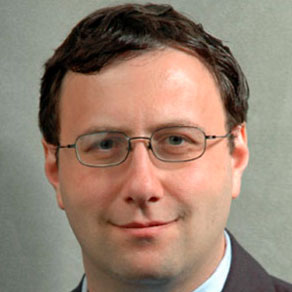
Mikhail Belkin
[intermediate/advanced] Modern Machine Learning and Deep Learning through the Prism of Interpolation
Summary
In the past decade the mathematical theory of machine learning has lagged far behind the triumphs of deep neural networks on practical challenges. However, the gap between theory and practice is gradually starting to close. I will attempt to assemble some pieces of the remarkable and still incomplete mathematical mosaic emerging from the efforts to understand the foundations of deep learning. The two key themes will be interpolation, and its sibling, over-parameterization. Interpolation corresponds to fitting data, even noisy data, exactly. Over-parameterization enables interpolation and provides flexibility to select a right interpolating model. As we will see, just as a physical prism separates colors mixed within a ray of light, the figurative prism of interpolation helps to disentangle generalization and optimization properties within the complex picture of modern machine learning.
Syllabus
The lectures will discuss various aspects of generalization and optimization in deep learning from the perspective of interpolation.
References
The lectures will be based on:
Fit without fear: remarkable mathematical phenomena of deep learning through the prism of interpolation. Mikhail Belkin, Acta Numerica, 2021 (https://arxiv.org/abs/2105.14368).
Pre-requisites
Basic knowledge of machine learning.
Short bio
Mikhail Belkin received his Ph.D. in 2003 from the Department of Mathematics at the University of Chicago. His research interests are in theory and applications of machine learning and data analysis. Some of his well-known work includes widely used Laplacian Eigenmaps, Graph Regularization and Manifold Regularization algorithms, which brought ideas from classical differential geometry and spectral analysis to data science. His recent work has been concerned with understanding remarkable mathematical and statistical phenomena observed in deep learning. This empirical evidence necessitated revisiting some of the basic concepts in statistics and optimization. One of his key recent findings is the “double descent” risk curve that extends the textbook U-shaped bias-variance trade-off curve beyond the point of interpolation. Mikhail Belkin is a recipient of a NSF Career Award and a number of best paper and other awards. He has served on the editorial boards of the Journal of Machine Learning Research, IEEE Pattern Analysis and Machine Intelligence and SIAM Journal on Mathematics of Data Science.