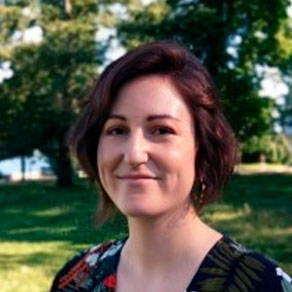
Emma Tolley
Physics-Informed Deep Learning
Summary
Next-generation scientific infrastructures will produce large volumes of data with unprecedented precision and resolution, but the analysis of these data and the generation of corresponding simulations pose significant computational challenges. While deep neural networks have been increasingly used to accelerate and automate data analysis and simulation development, they suffer from interpretability and generalization problems. However, prior knowledge can be used to inform the network architecture and training, greatly enhancing network reliability. Additionally, a new class of neural networks which encapsulate additional physics laws can allow for the development of accurate networks which require little to no training data. In this talk I cover strategies for incorporating prior knowledge into deep neural networks, with a focus on applications in radio astronomy and cosmological simulations.
Short bio
Emma Tolley is a staff scientist at the École polytechnique fédérale de Lausanne (EPFL) and the Data Science program chair of the Swiss SKA Consortium. She received her Bachelor’s degree in Physics from MIT in 2011 and completed her PhD in Physics at Harvard University in 2016. Her research includes leveraging data science techniques for the efficient, automated, and reliable computation and analysis of the Big Data produced by large scientific infrastructures such as CERN and the upcoming Square Kilometer Array Observatory.