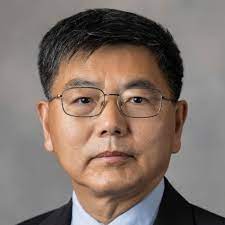
Lei Xing
[intermediate] Deep Learning for Medical Imaging and Genomic Data Processing: from Data Acquisition, Analysis, to Biomedical Applications
Summary
Artificial intelligence driven by deep learning has attracted much attention in the past decade and holds significant potential for improved healthcare. The enormous success of deep learning stems from its unique capabilities of extracting and aggregating essential features from big data and then making inferences. In my lectures, I will summarize recent advances in AI applications in imaging and therapy, interpretable AI, as well as genomics applications. I will also identify the pitfalls of current data-driven approaches and provide my perspectives on the future of AI in biomedical physics and medicine.
Syllabus
- Brief summary of medical imaging and its important roles in clinical practice.
- Highlights of different types of imaging techniques and their pro and cons.
- Multimodality and multiplex imaging.
- Roles of AI/deep learning in image analysis and highlights of a number of applications (segmentation, registration, reconstruction).
- Examples: data-driven sparse image reconstructions for CT and MRI.
- Examples: AI applications in radiation therapy treatment planning.
- Examples: Data-driven therapeutic response.
- Summary of genomic techniques and traditional high dimensional data analysis techniques.
- Single cell genomics and data processing.
- Summary.
References
1. Xing L, Giger ML, Min JK (editors): Artificial Intelligence in Medicine: Technical Basis and Clinical Applications. Academic Press, London, UK, 2020.
2. Zhang A, Xing L, Zou J, Wu J: Shifting machine learning for healthcare from development to deployment and from models to data. Nature Biomedical Engineering, appeared online. DOI 10.1038/s41551-022-00898-y, 2022.
3. Shen L, Pauly J, Xing L, NeRP: Implicit Neural Representation Learning with Prior Embedding for Sparsely Sampled Image Reconstruction. IEEE Transactions on Neural Networks and Learning Systems, appeared online, 10.1109/TNNLS.2022.3177134, 2022.
4. Islam MT, Xing L: A data-driven dimensionality-reduction algorithm for the exploration of patterns in biomedical data. Nature Biomedical Engineering, 5: 624-635, 2021.
5. Seo H, Bassenne M, Xing L: Closing the Gap between Deep Neural Network Modeling and Biomedical Decision-Making Metrics in Segmentation via Adaptive Loss Functions, IEEE Transactions on Medical Imaging, 40: 585-93, 2021.
Pre-requisites
Basics of medical imaging. Basic understanding of machine learning/deep learning/convolutional neural networks. Basic knowledge of data science.
Short bio
Dr. Lei Xing is currently the Jacob Haimson & Sarah S. Donaldson Professor and Director of Medical Physics Division of Radiation Oncology Department at Stanford University. He also holds affiliate faculty positions in the Department of Electrical Engineering, Biomedical Informatics, Bio-X and Molecular Imaging Program at Stanford (MIPS). He has been a member of the Radiation Oncology faculty at Stanford since 1997. Dr. Xing obtained his PhD in Physics from the Johns Hopkins University in 1992 and received his Medical Physics training at the University of Chicago. His research has been focused on artificial intelligence in medicine, treatment planning and dose optimization, medical imaging, imaging instrumentations, image guided interventions, nanomedicine, and applications of molecular imaging in radiation oncology. Dr. Xing is an author on more than 400 peer reviewed publications, a co-inventor on many issued and pending patents, and a co- investigator or principal investigator on numerous NIH, DOD, NSF, RSNA, AAPM, Komen, ACS and corporate grants. He is a fellow of AAPM (American Association of Physicists in Medicine) and AIMBE (American Institute for Medical and Biological Engineering).