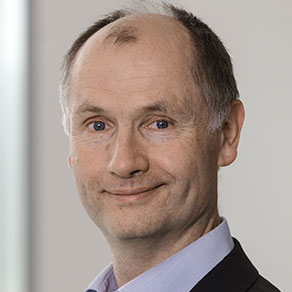
Martin Schultz
[intermediate] Deep Learning for Air Quality, Weather and Climate
Summary
The environment we live in is a complex system with manifold feedbacks across a wide range of spatial and temporal scales. Environmental sensors generate massive amounts of data every day, and numerical models are employed around the world to predict air quality, weather, and climate. Deep learning has many potential applications in the weather, climate, and air quality domain. Beyond forecasting, it can be used to assist in the consistent processing of observations (data assimilation), to improve empirical parameterisations in numerical models, to generate enhanced output through better post-processing, and more. While the chaotic behaviour of the climate system imposes large challenges on deep learning approaches, the recent large-scale models seem to overcome these with astonishing quality. Recently, a number of ground-breaking studies showed that deep learning, which has been so successful in areas such as image or speech recognition or robotics, has a huge potential to improve our current predictive capabilities, or at the least to achieve similar results at much cheaper computational cost. This has important implications for several policy and economic sectors and for the atmospheric science community. Only three years ago, it seemed like a long journey for deep learning to approach the state-of-the-art in weather modelling. Now, there are indications that deep learning models might generate even more reliable forecasts, although more careful evaluation is still needed. The course will begin with an introduction to weather and climate from a data science perspective. We will then summarize the history of machine learning in this area and compare it with traditional numerical modelling techniques. In the third part, we will discuss the recent state-of-the-art literature based on large-scale transformer-based models.
Syllabus
- Part 1: Introduction to weather and climate from a data science perspective
- Part 2: Machine learning versus numerical modelling of air quality, weather and climate
- Part 3: Current research into deep learning for air quality, weather and climate
References
Bi, Kaifeng, Lingxi Xie, Hengheng Zhang, Xin Chen, Xiaotao Gu, und Qi Tian. “Pangu-Weather: A 3D High-Resolution Model for Fast and Accurate Global Weather Forecast”. arXiv, 3. November 2022. http://arxiv.org/abs/2211.02556.
Lam, Remi, Alvaro Sanchez-Gonzalez, Matthew Willson, Peter Wirnsberger, Meire Fortunato, Alexander Pritzel, Suman Ravuri, u. a. “GraphCast: Learning Skillful Medium-Range Global Weather Forecasting”. arXiv, 24. December 2022. http://arxiv.org/abs/2212.12794.
Nguyen, Tung, Johannes Brandstetter, Ashish Kapoor, Jayesh K. Gupta, und Aditya Grover. “ClimaX: A foundation model for weather and climate”. arXiv, 24. January 2023. https://doi.org/10.48550/arXiv.2301.10343.
Pathak, Jaideep, Shashank Subramanian, Peter Harrington, Sanjeev Raja, Ashesh Chattopadhyay, Morteza Mardani, Thorsten Kurth, u. a. “FourCastNet: A Global Data-driven High-resolution Weather Model using Adaptive Fourier Neural Operators”. arXiv:2202.11214 [physics], 22. February 2022. http://arxiv.org/abs/2202.11214.
Reichstein M., Camps-Valls G., Stevens B., Jung M., Denzler J., Carvalhais N., et al. 2019. Deep learning and process understanding for data-driven earth system science. Nature 566(7743), 195–204. (doi:10.1038/s41586-019-0912-1)
Schultz M. G., Betancourt C., Gong B., Kleinert F., Langguth M., Leufen L. H., Mozaffari A. and Stadtler S. (2021): Can deep learning beat numerical weather prediction? Phil. Trans. R. Soc. A.3792020009720200097. http://doi.org/10.1098/rsta.2020.0097.
Pre-requisites
Good knowledge of basic machine learning (linear regression, clustering, random forest, etc.); some knowledge of deep learning methods (FCN, CNN, Auto-encoder, LSTM, GAN); basic knowledge of statistics; fascination about the atmosphere.
Short bio
Martin Schultz is a senior researcher at the Jülich Supercomputing Centre, Germany. After a successful career as environmental scientist developing air pollution and chemistry climate models, he started a new line of research to investigate how deep learning can be used to improve the analysis and predictions of air quality, weather and climate. Through the ERC Advanced Grant IntelliAQ which was awarded to him in 2018 and through several other research projects, Schultz built a 20-member team of atmospheric scientists, computer scientists and mathematicians who adopt state-of-the-art deep learning models for atmospheric applications, develop new approaches, and analyse the fundamental properties of the data and the models in order to understand why certain methods work better than others and where the theoretical limitations of deep learning may be. His research group also develops scalable, performant machine learning workflows and software packages and is involved in the creation of benchmark datasets to help boost deep learning developments in environmental research. Schultz has been listed twice as highly cited researcher and has given several courses on atmospheric science and, more recently, machine learning.