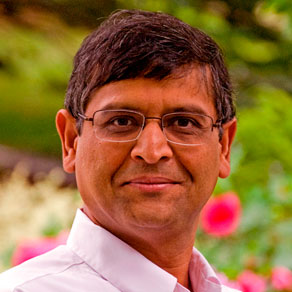
Vipin Kumar
Knowledge-Guided Deep Learning: A Framework for Accelerating Scientific Discovery
Summary
Process-based models of dynamical systems are often used to study engineering and environmental systems. Despite their extensive use, these models have several well-known limitations due to incomplete or inaccurate representations of the physical processes being modeled. There is a tremendous opportunity to systematically advance modeling in these domains by using state of the art deep learning methods that have already revolutionized computer vision and language translation. However, “black box” deep learning methods often lead to models that are not generalizable beyond the samples used for training. This problem becomes worse when there is a scarcity of labeled samples, which is quite common in science and engineering domains. This talk makes the case that in real-world systems that are governed by physical processes there is an opportunity to take advantage of fundamental physical principles to inform the search of a physically meaningful and accurate machine learning model. While this talk will illustrate the potential of the knowledge-guided deep learning paradigm in the context of environmental problems (e.g., Fresh water science, Hydrology, Agronomy), the paradigm has the potential to greatly advance the pace of discovery in a diverse set of disciplines where mechanistic models are used, e.g., climate science, weather forecasting, and pandemic management.
Short bio
Vipin Kumar is a Regents Professor and holds William Norris Chair in the department of Computer Science and Engineering at the University of Minnesota. Kumar’s current major research focus is on knowledge-guided machine learning and its applications to understanding the impact of human induced changes on the Earth and its environment. He has authored over 400 research articles, and co-edited or co-authored 11 books, including two widely used text books “Introduction to Parallel Computing”, “Introduction to Data Mining”, and a recent edited collection, “Knowledge Guided Machine Learning”. Kumar is a Fellow of the AAAI, AAAS, ACM, IEEE, and SIAM. Kumar’s foundational research in data mining and high performance computing has been honored by the ACM SIGKDD 2012 Innovation Award, which is the highest award for technical excellence in the field of Knowledge Discovery and Data Mining (KDD), the 2016 IEEE Computer Society Sidney Fernbach Award, one of IEEE Computer Society’s highest awards in high performance computing, and Test-of-time award from 2021 Supercomputing conference (SC21).