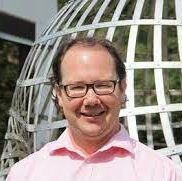
Holger Rauhut
[intermediate] Gradient Descent Methods for Learning Neural Networks: Convergence and Implicit Bias
Summary
Gradient descent and stochastic gradient descent methods are at the core of training deep neural networks. Due to non-convexity of the loss functional and overparameterization convergence properties of these methods are not yet well-understood. This lecture series aims at introducing to mathematical aspects of learning deep neural networks and presenting initial results for simplified cases.
After a general introduction to (stochastic) gradient descent methods for deep learning, we will focus on linear neural networks (i.e., with linear activation function) for the theoretical analysis. While linear networks are not expressive enough for most applications, their mathematical analysis still poses significant challenges which should be understood before passing to nonlinear networks. Instead of (stochastic) gradient descent (SGD) methods, it is also beneficial to first study the corresponding gradient flow, which avoids a discussion of step size choices. We will show convergence to critical points of the loss functional, and for the square loss show convergence to global minima (both for the gradient flow and gradient descent). Moreover, the factorization structure of linear networks induces a Riemannian geometry so that the flow of the network can be interpreted as a Riemannian gradient flow.
In many learning scenarios one uses significantly more neural network parameters than training data. Despite the fact that then many networks exist which interpolate the data exactly so that the loss functional has many global minimizers, learned neural networks generalize very well to unseen data, which is in contrast to intuition from classical statistics that such a scenario would lead to overfitting. The used learning algorithms, i.e., (stochastic) gradient descent (SGD) methods (together with their initialization) impose an implicit bias on which minimizer is computed. The implied bias of (S)GD seems to be very favorable in practice. A working hypothesis is that (S)GD with small initialization promotes low complexity in a suitable sense. We will present first mathematical results in this direction for linear networks, where sparsity and/or low rank is promoted.
Syllabus
- Introduction to training deep networks
- Convergence theory for gradient flow and gradient descent for linear neural networks
- Mathematical analysis of the implicit bias of gradient flow and gradient descent for learning linear neural networks in overparameterized scenarios
References
S. Arora, N. Cohen, N. Golowich, and W. Hu. A convergence analysis of gradient descent for deep linear neural networks, ICLR, 2019. arXiv:1810.02281.
S. Azulay, E. Moroshko, M. S. Nacson, B. Woodworth, N. Srebro, A. Globerson, and D. Soudry. On the implicit bias of initialization shape: Beyond infinitesimal mirror descent, 2021. arXiv:2102.09769.
B. Bah, H. Rauhut, U. Terstiege, M. Westdickenberg. Learning deep linear neural networks: Riemannian gradient flows and convergence to global minimizers. Information and Inference, Volume 11, Issue 1, 2022, pp 307–353.
G. M. Nguegnang, H. Rauhut, U. Terstiege. Convergence of gradient descent for learning linear neural networks. Preprint, 2021. arXiv:2108.02040.
H.-H. Chou, C. Gieshoff, J. Maly, H. Rauhut. Gradient Descent for Deep Matrix Factorization: Dynamics and Implicit Bias towards Low Rank. Preprint, 2020. arXiv:2011.13772.
H.-H. Chou, J. Maly, H. Rauhut. More is Less: Inducing Sparsity via Overparameterization. Preprint, 2022.
B. Neyshabur, R. Tomioka, and N. Srebro. In search of the real inductive bias: On the role of implicit regularization in deep learning. ICLR, 2015.
F. Wu and P. Rebeschini. Implicit regularization in matrix sensing via mirror descent. Advances in Neural Information Processing Systems, 34, 2021.
C. Zhang, S. Bengio, M. Hardt, B. Recht, and O. Vinyals. Understanding deep learning requires rethinking generalization. ICLR, 2017.
Pre-requisites
Multivariate analysis. Linear algebra. Basic knowledge on optimization helpful, but not necessary.
Short bio
1996 – 2001 Study of Mathematics at Technical University of Munich
2002 – 2004 Doctoral studies in Mathematics, Technical University of Munich (Supervisor: Prof. Dr. Rupert Lasser)
2005 – 2008 Postdoc at University of Vienna, Numerical Harmonic Analysis Group (Mentor: Prof. Dr. Hans Feichtinger)
2008 Habilitation in Mathematics
2008 – 2013 Professor of Mathematics (“Bonn Junior Fellow”) at University of Bonn, Hausdorff Center for Mathematics
Since 2013 Professor of Mathematics, RWTH Aachen University, Chair for Mathematics of Information Processing
2016 – 2018 Head of Department of Mathematics, RWTH Aachen University
2018 – 2022 Member of the Senate, RWTH Aachen University
Since 2022 Spokesperson of Collaborative Research Center “Sparsity and Singular Structures” (SFB 1481)