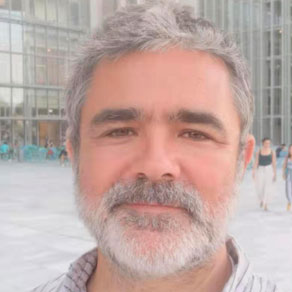
Michalis Vazirgiannis
[intermediate/advanced] Graph Machine Learning with GNNs and Applications
Summary
Graphs are abundant and dominate a large group of applications as natural data structures representing more effectively the knowledge inherent in data. In this mini course we will present initially the challenge of Graph Machine Learning, the concept of graph similarity via graph kernels with applications such as community detection and impact evaluation. Also an introduction to the Grakel python library enabling machine learning with kernels. Then we move to deep learning for graphs with a mild introduction of Graph Neural Networks (GNN) with node and graph embedding algorithms along with relevant experimental evaluation tasks. We put emphasis on the message passing GNN model but also present alternatives such as the RWNN – enabling explainability, Hyperbolic Graph Neural Networks for better hierarchical relations treatment etc. We also present an introduction to the topic of generative models for graphs with emphasis in GVAEs. We also touch upon the topic of multimodality for graphs. Finally we present novel applications of GNNs for down stream and generative tasks including NLP and medicine (antibiotic resistance prediction, medical data generation).
Syllabus
1. Graph Similarity
- Graph kernels, community detection
- Grakel python library – https://github.com/ysig/GraKeL/
2. Deep Learning for Graphs – Node classification
- Node embeddings (deepwalk & node2vec) for node classification and link prediction
- Supervised node embeddings (GCNN, …)
3. Deep Learning for Graphs – Graph classification
- Graph CNNs
- Message passing, popular GNNs
4. Applications of GNNs
- Natural language – Document understanding
- Bio/medical (ARG prediction)
- Recommendations
- Time series predictions
5. Generative and Pretrained Models for Graphs
- Pretrained and generative models (GVAEs)
- Multimodality with graphs
- Applications (medical graphs generation)
References
Graph Similarity
- Graph kernels: A survey, G. Nikolentzos, G. Siglidis, M. Vazirgiannis, Journal of Artificial Intelligence Research 72, 943-1027.
- Learning Structural Node Representations on Directed Graphs, N. Steenfatt, G. Nikolentzos, M. Vazirgiannis, Q. Zhao, International Conference on Complex Networks and their Applications, 132-144.
- Classifying graphs as images with convolutional neural networks, A.J.P. Tixier, G. Nikolentzos, P. Meladianos, M. Vazirgiannis, ICANN 2018.
Deep Learning for Graphs
- F. Scarselli, M. Gori, A. C. Tsoi, M. Hagenbuchner, and G. Monfardini. The Graph Neural Network Model. IEEE Transactions on Neural Networks, 20(1):61–80, 2009.
- Y. Li, D. Tarlow, M. Brockschmidt, and R. Zemel. Gated Graph Sequence Neural Networks. arXiv preprint arXiv:1511.05493, 2015.
- J. Gilmer, S. S. Schoenholz, P. F. Riley, O. Vinyals, and G. E. Dahl. Neural Message Passing for Quantum Chemistry. In Proceedings of the 34th ICML conference, pp. 1263–1272, 2017.
- M. Zhang, Z. Cui, M. Neumann, and Y. Chen. An End-to-End Deep Learning Architecture for Graph Classification. In Proc. 32nd AAAI Conference on Artificial Intelligence, pp. 4438–4445, 2018.
- C. Morris, M. Ritzert, M. Fey, W. L. Hamilton, J. E. Lenssen, G. Rattan, and M. Grohe. Weisfeiler and Leman Go Neural: Higher-order Graph Neural Networks. In Proceedings of the 33rd AAAI Conference on Artificial Intelligence, 2019.
- K. Xu, W. Hu, J. Leskovec, and S. Jegelka. How Powerful are Graph Neural Networks? In Proceedings of the 7th International Conference on Learning Representations, 2019.
- Diffusion Convolutional Recurrent Neural Network: Data-Driven Traffic Forecasting, Yaguang Li, Rose Yu, Cyrus Shahabi and Yan Liu, https://arxiv.org/pdf/1707.01926.pdf.
- Spectral Temporal Graph Neural Network for Multivariate Time-series Forecasting, Defu Cao, Yujing Wang, Juanyong Duan, Ce Zhang, Xia Zhu, Conguri Huang, Yunhai Tong, Bixiong Xu, Jing Bai, Jie Tong and Qi Zhang, https://arxiv.org/pdf/2103.07719.pdf.
Generative and Pretrained Models for Graphs
- Yuanfu Lu, Xunqiang Jiang, Yuan Fang, Chuan Shi, Learning to Pre-train Graph Neural Networks, AAAI 2021.
- Aymen Qabel, Sofiane Ennadir, Giannis Nikolentzos, Johannes F. Lutzeyer, Michail Chatzianastasis, Henrik Boström, Michalis Vazirgiannis, Structure-Aware Antibiotic Resistance Classification Using Graph Neural Networks, https://www.biorxiv.org/content/biorxiv/early/2022/10/08/2022.10.06.511103.full.pdf.
- Synthetic electronic health records generated with variational graph autoencoders, Giannis Nikolentzos, Michalis Vazirgiannis, Christos Xypolopoulos, Markus Lingman, Erik G. Brandt, https://www.medrxiv.org/content/10.1101/2022.10.17.22281145v1.
Pre-requisites
Good understanding of algorithms, graphs and machine and deep learning.
Short bio
Dr. Vazirgiannis is a Distinguished Professor at École Polytechnique, Institut Polytechnique de Paris in France. He has conducted research in Fraunhofer and Max Planck-MPI (Germany), in INRIA/FUTURS (Paris). He has been teaching data mining, machine and deep learning in AUEB (Greece), École Polytechnique, Telecom-Paristech, ENS (France), Jiaotong Shanghai (China) and in Deusto University (Spain). His current research interests are on graph machine/deep learning, including GNNs, community detection, graph classification, clustering and embeddings, influence maximization. Recently he is interested in multimodal pretrained models for downstream and generative tasks. Also he has long experience in text mining including graph of words, deep learning for NLP tasks and applications such as digital marketing, event detection and summarization. He has active cooperations with industrial partners in the area of data analytics and machine learning for large scale data repositories in different application domains. He has supervised more than twenty five completed PhD theses, has published three books and more than 250 papers in international refereed journals and conferences and received best paper (or mention) awards in ACM CIKM 2013 and IJCAI 2018, ICWSM. He has organized large scale conferences in the area of data mining and machine learning (such as ECML/PKDD) while he participated in the senior PC of AI and ML conferences – such as AAAI and IJCAI. He has received the ERCIM and the Marie Curie EU fellowships, the Rhino-Bird International Academic Expert Award by Tencent and he lead(s) important chairs such as: AXA Data Science (2015 – 2018), the ANR-HELAS (2020-25) and WASP/KTH (2020-2025). More information at the DASCIM web page: http://www.lix.polytechnique.fr/dascim and Google Scholar profile: https://bit.ly/2rwmvQU.